


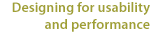

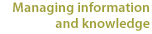


 |
Knowledge Management in
Pursuit of Performance: the Challenge of Context
by
Duane Degler and Lisa Battle
Performance Improvement, ISPI, 39(6), July 2000
Read our additional
thoughts on the subject, March 2002
The
rabbit-hole went straight on like a tunnel for some way, and then
dipped suddenly down, so suddenly that Alice had not a moment to think
about stopping herself before she found herself falling down what
seemed to be a very deep well.
Lewis
Carroll, Alice in Wonderland
Introduction
Knowledge
Management (KM) has an increasingly visible profile within
organizations, in part because of the growing use of intranets for
communication and information retrieval. It has long been recognized
that sharing knowledge and experience among people has the potential
to improve the quality of work and the quality of decisions that are
made – and the discipline of knowledge management has arisen to
realize this value over increasing spans of time and distance.
However,
the popularity of KM has led to some confusion in the industry about
the difference between knowledge and information (Huang, Lee &
Wang 1999; Glazer 1999). Much of the current knowledge management
focus has been on the acquisition and storage of “knowledge
resources,” although one of the principal values of KM has always
been communication between individuals as a way to improve knowledge
transfer (Nonaka & Takeuchi 1995; Davenport & Prusak 1998).
Many knowledge management projects have created stand-alone
information bases. While such repositories are a good start, they do
not go far enough. Finding information is not the same thing as
applying knowledge. It is not an end in itself.
This
article explores opportunities for taking the next step and
integrating KM seamlessly into business applications. What would
applications be like if they were tightly coupled with KM? Information
bases would exist, but the user would not need to interact with them
directly. In an ideal world, business applications (such as inventory
management, purchasing, and correspondence routing systems) would be
designed to support the goals of the work, and users would be able to
focus on doing their own work using the application as a tool.
Whenever information could improve the outcome of the work, the
application would identify the need, ask the information base for
information, receive the information, package it, and present it to
the users in whatever format was most appropriate. The users would get
what they needed, whether they knew they needed it or not, and
continue to work without interruption. The whole process of gathering
information would normally be invisible to the users.
Of
course, we know that this does not happen today. Most of the time,
gathering information is painfully visible to the user (Frappaolo
1998; Henniger & Belkin 1994; Blair & Maron 1985).
Often users have to leave their work and launch another
application to seek information.
They may have to type a syntactically correct query into a
search engine that retrieves a long list of documents that may or may
not be relevant. They then start a different
search within each identified document to find data – or an idea –
that helps them. It
takes a lot of effort to describe the need and to evaluate the
results.
As
long as KM settles for stand-alone information bases that are not
closely linked with business applications, it will not live up to its
potential. As Michael Schrage recently said, "Knowledge is not
the power. Power is power. The ability to act on knowledge is
power" (Gurteen 1999). There is a need to focus on achieving
successful performance outcomes. This is already the focus of
Performance-Centered Design (PCD), an approach to designing business
applications which has gained popularity in recent years. PCD
applications typically aim to help inexperienced or infrequent users
complete tasks successfully -- as if they were experts -- and to
increase the overall quality of work products. The interfaces are
easier to learn and use because they clearly match the goals and work
processes of their users and provide timely information. However, many
current designs rely on an information base that is relatively simple
and static and tasks that are clearly specified.
This does not work as well when designing for complex, changing
environments or for knowledge workers whose responsibilities are less
structured. The time has come to combine and extend the current PCD
and KM approaches, with an emphasis on applying knowledge to support
successful business outcomes.
Applying
knowledge to support business outcomes
“‘Knowledge’
is generated when information is combined with context and
experience.”
(Huang, Lee and Wang,
1999, p146)
In
business terms, knowledge is reflected in the quality of an outcome
– whether the task is operating machinery or deciding strategic
direction. Knowledge
benefits the organization when it is applied. Therefore, in order to
reap the benefits, we need to find ways of bringing context,
experience, and information into business applications to support
successful performance outcomes.
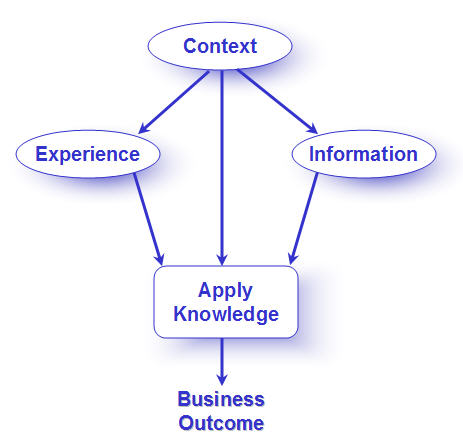
Figure 1. Contributors to successfully applying knowledge.
Historically,
PCD has emphasized the context in which a person performs and, through
such mechanisms as role-based views and task-oriented interfaces, has
attempted to provide "just enough, just in time" information
appropriate to the context. KM practice has grown out of two main
areas: information management (the acquisition, storage and
dissemination of structured and unstructured information) and
communication (represented as experience because the main goal of communication between people in
KM terms is the sharing of experience to increase knowledge). We will
briefly explore context, experience, and information in turn before
going on to look at techniques for incorporating them into business
applications.
Context
Context
is the collection of relevant conditions and surrounding influences
that make a situation unique and comprehensible. The human cognitive
and perceptual systems are designed to identify and use context
automatically as we go about our daily lives (Anderson 1995; Hasher
& Zacks 1984). It is harder than originally imagined to teach a
machine to do the same. The field of Artificial Intelligence has been
exploring the implications of context in areas such as natural
language processing for decades and has had to readjust its initial
high hopes for machines that can mimic human intelligence (Ashcraft
1994). Context is enormously complex. It involves numerous interacting
factors that people do not necessarily even pay attention to on a
conscious level, and many of which are outside the ability of machine
input devices to capture.
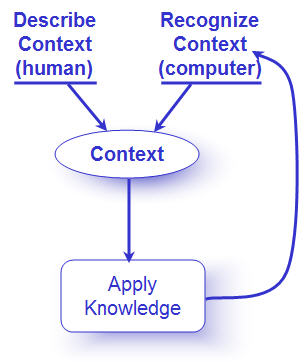
Figure 2. Building a relevant view of business context.
There
are two approaches to identifying context in business applications.
Combining these approaches so that some attributes of context are
drawn from the individual (description) and some from the computer
(recognition) is often the best solution.
Context
description
means the person provides details that are outside the computer
environment, particularly any implicit/subjective conditions. The way
that someone describes context depends on how much they know about the
subject, what they perceive to be the problem, and what kind of
facilities they are given to describe it. Business applications should
become more proactive in eliciting context from users in situations
where the users need to ask for information.
Context
recognition
is an automated approach to context. Business applications should be
familiar with their users, business processes, and subject domains.
When the user asks for information, the application should be able to
identify and bring forward the knowledge resource that best
corresponds to the current context. When the user does not ask, the
application should provide information anyway if necessary to achieve
a successful outcome. A
good understanding of context (on the application's part) is
especially crucial in this situation, because "push"
information and feedback can be extremely frustrating to the user if
it is not relevant – the result being that the user ignores the
information or turns the feature off entirely.
Experience
Identifying
and describing context are not enough, of course. The user also needs to have a mental model of how to approach
the task at hand. This typically comes from experience. There are
three broad areas of experience that support successful performance.
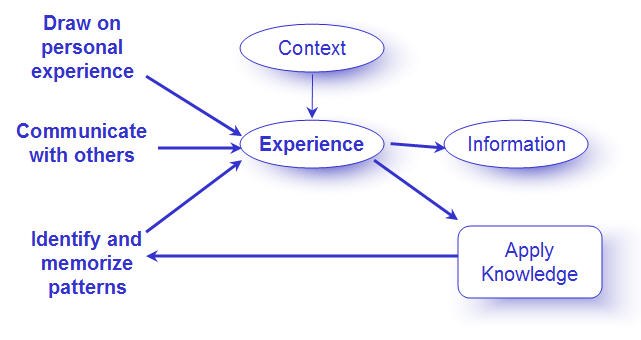
Figure 3. Drawing on a wide range of experience.
Individual
experience:
An individual draws on personal experience both within the subject domain
and outside of it. The depth of experience will affect the
individual's approach to problem-solving and decision-making. Research
in cognitive psychology has shown significant differences between
novices and experts in the way they think (Tanaka
& Taylor, 1991). For
example, an expert has an enhanced ability to categorize circumstances
and an extensive set of schemas for problem-solving, which helps
mentally structure the need for additional information, but may also
restrict the degree to which new information is sought (Feltovich et
al., 1997).
Others’
experience:
An individual can also call on other people's experience. Connecting
with other people has a number of advantages. It allows for knowledge
to be passed from person to person, which benefits the organization.
It also increases the possibility that information and experiences are
translated into tangible form – such as written in a document by one
person for another – that can become part of the overall information
available in the organization. Also, it challenges both individuals to
unmask assumptions and misconceptions that can become a barrier.
Collective
memory and patterns:
If an application is able to capture
best practices and patterns of expert behavior based on successful
performance, then that information can be used as a model for future
performance. The application can compare a current situation with a
pattern of best practice to determine whether the user is "on
track." If not, the application can provide suggestions
based on the collective experience of the group of users.
Information
The
quality of information depends on its accuracy and its relevance to
the context. Some valuable information management approaches, outside
the scope of this article, are being explored in both KM and PCD
disciplines (Yacci 1999). The work that is being done needs to be
shared and enhanced to meet the growing needs of the merged
disciplines. Areas of investigation include:
-
granularity of
information – both in terms of storage and presentation
-
the importance of
metadata, particularly as it relates to context
-
increasing the
reusability of information, including the creation of ‘knowledge
objects’
-
structuring
information in ways that move away from the ‘document’ and 'page'
metaphors
The
ideal PCD/KM application should provide information that is both
accurate and relevant without requiring the user to actively seek this
information and determine its relevance. This will prevent information
seeking from becoming a complex problem-solving activity of its own.
Techniques
for merging PCD and KM
By
bringing context, experience, and information together, we can begin
to create more powerful, flexible, performance-centered business
applications that support successful outcomes. These applications will
assist the user by integrating dynamic knowledge repositories into the
work environment, facilitating access to peers and experts as needed,
and allowing both the human and the computer to do what they do best
to achieve a business outcome. We have been exploring techniques for
doing this, including:
-
Multi-layer context profiles
-
Proactive context-based information retrieval
-
Stealth knowledge management
-
Adaptive knowledge interfaces.
1.
Multi-layer context profiles
To
achieve any real benefits from automated context recognition,
designers need to look for better ways of identifying context. We
propose that context has multiple layers that overlap and intersect
when describing a situation. These layers may include:
-
The user's current activities in the application
-
The user's role, background, working patterns,
experience with the subject domain, previous use of information, and
experience with relevant problem-solving or information-seeking
strategies
-
Business rules, policies and processes, including
aspects that are time-dependent (e.g. “this action must be completed
every Thursday before 3pm”)
-
Patterns of use in the user's peer community, including
what other people have done before in this context
-
System conditions, such as what other applications are
running, the historic record of actions that led up to the current
situation, what information resources are available and how recently
the resources have been updated
-
Environmental conditions, including who else is working
in the same subject domain, whether they are logged on, whether the
current time is within their normal working hours, and whether they
have other appointments (to determine whether people are able to be
contacted to share their knowledge)
Techniques
already exist for capturing much of this contextual information, but
each piece of it comes from a different source, and they are not
combined to achieve this kind of precision. It is increasingly
important to do so. All
the above information allows relevant knowledge to be provided to the
individual and to be elicited from the individual for use by the
organization. It also
allows a dynamic PCD application and interface to effectively meet the
needs of the user and the task being performed.
For
example, multi-layer context profiles are being explored in the design
of an inventory system for a large art sales event. Sales staff with a
wide range of backgrounds and experience levels must be able to
clearly inform potential buyers about both the work and the artist.
Sales staff only approach the computer when a question arises – they
do not regularly use the application. Ideally the inventory system
should provide concise and accurate information while reflecting the
experience and selling style of the salesperson.
It could recognize and respond to:
-
User's domain knowledge (e.g. a ceramics expert will
need more support to answer questions about 19th century
oils)
-
User's past usage patterns (e.g. when talking with a
potential buyer, one salesperson typically retrieves details on the
associated school/style, while another prefers to see comparisons to
similar artworks that he has learned about previously)
-
Time-dependent factors (e.g. if the event is currently
in progress, it may check whether the item has been sold yet and
recommend similar works to the buyer)
-
Geographical location of the event (e.g. at point of
purchase, listings of local/regional information sources can be
accessed based on the item purchased)
2.
Proactive
Context-Based Information Retrieval
Sometimes
a knowledge worker needs access to information from multiple sources
as part of a decision-making process. Instead of requiring the user to
search each source individually, the business application can provide
proactive context-linked information retrieval. This approach is
proactive because it is initiated by the application, not the user. It
is linked to the context because the facts of the current case trigger
the retrieval of relevant information (including data, policy, and
reference materials). The application performs the search in the
background and presents a summary of its findings or relevant links
embedded in the interface at appropriate points in the work context.
For
example, for an insurance company's claims management system, we
designed an interface to reflect each phase of analysis when handling
insurance claims, in order to provide information relevant to that
context. During each phase, the application would automatically
retrieve information from multiple reference sources, as well as from
past claims, and dynamically create links in appropriate places within
the electronic claim file. When the claims specialist was evaluating
medical information, the application would proactively identify
relevant medical tests and reference topics related to the claimant's
condition and the point in the decision-making process. The interface
would also identify any applicable state laws and retrieve lists of
previous cases with similar attributes to assist the claims specialist
in following best practice for handling the claim.
3.
Stealth Knowledge Management
We
are beginning to develop an approach we call
“Stealth Knowledge Management” – the ability to gain knowledge
of best practices by capturing the way people perform tasks.
The more computers are used to carry out job tasks and make
decisions, the more there is a growing record of the way decisions are
made – embodied in the way the applications are used. Unlike some
expert system and knowledge management approaches, the rules of
decision-making are not artificially defined in advance, but based on
actual performance. They
are as dynamic as the experienced people who perform the tasks day-in
and day-out. The
capturing of this experience (“knowledge from actions”) can be
valuable to less experienced people performing the same tasks.
The
key factors of Stealth Knowledge Management are:
-
the data is a representation of the business knowledge
of experienced users
-
the knowledge is dynamic: it changes as the business
context and application use change
-
decision-making patterns define rules that can be
modified or weighted based on the qualitative outcomes of the tasks
which generated the patterns
-
the knowledge can be made available immediately when a
decision is to be made, presented as recommendations for action
For
example, in one organization's correspondence routing system, all
requests for information from the public were channeled through a
central office. Experienced users in that office categorized the
requests and assigned them to other departments to respond. The
categorization and assignment of requests was a very subjective
process, and when a less experienced person had to take over (for
example, when primary staff were ill), they could not perform the
task. One design approach was to compare the request description with
the responses eventually received from the departments. The goal over
time was to create patterns of ‘probable suppliers’ of requested
information. When a user entered a new request, the application could
recommend departments, along with a ranking to indicate higher or
lower probabilities that they could successfully respond.
There were two keys to this concept:
-
The possible routing paths needed to be presented as
options (not as required rules for routing), because the user needed
the flexibility to evaluate subjective factors and make the final
routing decision
-
The application’s ranking system would constantly be
fed with every routing decision made, giving it the ability to
continue ‘learning’ over time.
The
Stealth Knowledge Management approach is particularly exciting due to
the invisibility of knowledge creation. This sort of application is a
strong candidate for tools that work in the background, monitoring
such things as message traffic between applications and the operating
system, because they can be applied to existing applications without
recoding. Also, the increasing availability of simple, plain-language
neural network and fuzzy logic tools to support pattern identification
and monitoring begin to make this a viable approach when developing
new applications.
4.
Adaptive Knowledge Interfaces
Providing
information relevant to the current context is not enough to ensure
successful performance. A person's ability to apply information
depends in part on his/her level of existing knowledge. Instead of
building one-size-fits-all interfaces, we may need to present
information differently to different users based on their individual
preferences and expertise. (This is similar in concept to
"adaptive interfaces" in which a first-time user sees a
limited set of features. Additional features become available later
and support features disappear as they are no longer required.)
A
system designed to do this would have both a knowledge layer and a
context-aware presentation layer, which would evaluate the user's
expertise and the work context when determining what information to
provide. The interface could adapt its:
-
Content detail: To avoid overwhelming
novices with detail, content could be regulated by identifying topics
as appropriate for beginners or experts and displaying them
accordingly.
-
Feedback style: An interface could
provide more detailed feedback and do so more frequently if the user
was unfamiliar with the subject domain, while an interface for experts
could display limited feedback. The application could evaluate
the business importance of the feedback, derived in part from
best practices, in determining whether or not to interrupt the user's
work.
-
Instruction: More instruction and
more explicit labels could be shown if needed to clarify unfamiliar
tasks. The instructional components of the interface could also be
enhanced for contexts that are rare or in situations where the user
appears to be deviating from best practices.
-
Structure and sequence: The
application could provide increasing levels of decision support and
structure for the work. It could help users with limited domain
knowledge to categorize the problem, structure the problem space, and
select the appropriate course of action, based in part on patterns and
best practices drawn from previous user experience. Providing
additional structure can help increase knowledge in the subject domain
while at the same time leading to a successful performance outcome.
For
example, in considering designs for an application to assist people
with developing and cataloguing classroom training, we realized that
some of the users were experienced instructors while others were
novice instructors who had been recruited to teach because of their
expertise in a subject area. The experienced instructors needed the
ability to create and organize their own training materials,
retrieving appropriate templates and checking the archives for
previous materials that could be used as starting points. The novice
instructors needed more structure to guide them through the process of
preparing for a course, but fewer background materials on the subject
itself. The interface for novices had to provide instructions on
preparing course objectives, the amount of material to cover in the
course, the appropriate types of exercises, what to include in
handouts, and so on. This more structured approach would help the
novice instructors to complete each step successfully.
Recognizing
the limits of automated support
Although
we have been talking about some of the advantages of automated context
recognition, we also think it is important to acknowledge the limits.
One of the things that KM brings to the merger is an understanding of
corporate knowledge as a dynamic thing. A KM system quickly becomes
obsolete if it does not continue to incorporate new information
quickly and does not have a mechanism for removing out-of-date or
contradictory information. This means
that making assumptions based on patterns of use will be tricky.
Patterns of use will change as circumstances change and knowledge
evolves.
The
implication for design is that a rigid system will frustrate the
knowledge worker who is trying to adapt to new circumstances. For
example, if the person who formerly advised on a subject has moved to
another position, a hard and fast rule for communication as part of a
knowledge management approach will create undue burdens. No matter how
good the underlying patterns and knowledge base, there will always be
a wrinkle that is not predicted.
Conclusion
Context
is the key to more invisibly and dynamically supporting business
performance – it is the area of greatest potential in the merger of
KM and PCD. If the two approaches can jointly rise to the challenge of
identifying context and responding appropriately, a great burden will
be lifted from the ordinary user.
What
we have come away from our experiences with are three simple rules:
-
Pay attention to the many facets of context if you want to provide
information to the knowledge worker.
-
Design applications that learn from everyone’s experience over time
– and keep learning.
-
Provide support as suggestions – not answers – in complex,
knowledge-rich environments so that the knowledge
worker remains in control.
Return to
the top of the page
References
Anderson,
J.R. (1995). Cognitive Psychology and its Implications, 4th edition.
New York: W.H. Freeman.
Ashcraft,
M.H. (1994) Human memory and
cognition. Second edition. New York: HarperCollins.
Blair,
D.C., & Maron, M.E. (1985). "An evaluation of retrieval
effectiveness for a full-text document-retrieval system." Communications
of the ACM, 28(3), 289-299.
Davenport,
T.H. & Prusak, L. (1998). Working
knowledge: how organizations manage what they know. Boston, MA:
Harvard Business School Press.
Feltovich,
P.J., Spiro, R.J., & Coulson, R.L. (1997). "Issues of expert
flexibility in contexts characterized by complexity and change."
In Feltovich, P.J., Ford, K.M., & Hoffman, R.R. (Eds.), Expertise
in Context, Menlo Park, CA: AAAI Press.
Frappaolo,
C. (1998). "Search and retrieval lay the foundation to knowledge
discovery." KMWorld, May 11, 1998.
Glazer,
R. (1998). "Measuring the knower: Towards a theory of knowledge
equity." Knowledge
Management 2(2), 12-18. Reprinted from the California Management Review 40(3).
Gurteen,
D. (1999). "The discipline of dialogue." Knowledge
Management 3(4), 30.
Hasher,
L., & Zacks, R.T. (1984). "Automatic processing of
fundamental information: the case of frequency of occurrence." American
Psychologist 39(12), 1372-1388.
Henniger,
S., & Belkin, N. (1994). "Interface issues and interaction
strategies for information retrieval systems." Proceedings
of CHI '94 (pp. 387- 338).
Huang,
K-T., Yang, W.L., & Wang, R.Y. (1999). Quality
Information and Knowledge. New Jersey: Prentice Hall.
Koulopoulos,
T. (1997). "The pieces of the knowledge management puzzle." KMWorld.
Lawton,
W. (1999). Electronic Performance Support Systems and Knowledge Management - The
Merging Ground. Dissertation, MSc Information Systems, University
of Brighton.
Nonaka,
I. & Takeuchi, H. (1995). The
knowledge-creating company: how Japanese companies create the dynamics
of innovation. New York: Oxford University Press.
Tanaka,
J. W., & Taylor, M. (1991). "Object categories and expertise:
is the basic level in the eye of the beholder?" Cognitive
Psychology, 23, 457-482.
Yacci,
M. (1999). "The Knowledge Warehouse: Reusing Knowledge
Components." Performance Improvement Quarterly, 12(3), 132-140.
Return to
the top of the page
Copyright, Reuse and
Citation
The content of this article may be referenced with the
appropriate citation information included (see below). The entirety of the article must
not be reproduced without written communication with ISPI (www.ispi.org).
Also, we would appreciate your notifying us if you intend to use these concepts or
images, as we are curious to know where they prove valuable.
To cite the material, please include the following
information. We recommend the format:
Degler, Duane and Battle, Lisa (2000). Knowledge
Management in Pursuit of Performance: the Challenge of Context. Performance
Improvement (EPSS Special Edition). ISPI, 39(6), July 2000. Online:
www.ipgems.com/writing/kmcontext.htm.
|